Overview
CNewSum is a large-scale Chinese news summarization dataset, which consists of 304,307 documents and human-written summaries from Toutiao. It is a extended version of TTNews for NLPCC2017 and NLPCC2018, which is much larger and has several features:
- The news article are collected from hundreds of thousands of news publishers. A team of expert editors are hired to provide human-written summaries for the daily news feed.
- Human-annotated labels are provided for each example in the test set to figure out how much knowledge the model needs to generate a human- like summary.
- Adequacy Level: Does necessary information of the summary has been included in the document?
- Deducibility Level: Can the information of the summary be easily inferred from the document?
We list the statistics of common English and Chinese summarization datasets. The 'Article' and 'Summary' are the average length of articles and summaries in the dataset. For English, it is calculated by words and for Chinese, it is calculated by characters.
Dataet | Train | Dev | Test | Total | Article | Summary | Source |
---|---|---|---|---|---|---|---|
NYT | 589.2k | 32.7k | 32.7k | 654.8k | 552.1 | 42.8 | New York Times |
CNNDM | 287.2k | 13.4k | 11.4k | 312.1k | 791.7 | 55.2 | CNN & Daily Mail |
Newsroom | 995.0k | 108.8k | 108.8k | 1.2m | 765.6 | 30.2 | 38 publishers |
LCSTS | 2.4m | 8.7k | 0.7k | 2.4m | 103.7 | 17.9 | |
RASG | 863.8k | - | - | 863.8k | 67.1 | 16.6 | |
TTNews | 50.0k | - | 4.0k | 54.0k | 747.2 | 36.9 | Toutiao |
CLTS | 148.3k | 20.3k | 16.7k | 185.3k | 1363.7 | 58.1 | ThePaper |
CNewSum | 275.6k | 14.4k | 14.4k | 304.3k | 730.4 | 35.1 | Toutiao |
We provide Coverage, Density and Compression to characterize our summarization dataset, which are introduced by Grusky et al.. We also compare n-gram novelties for Chinese characters.




We show one example for each Chinese summarization dataset. The overlaps between the article and the summary are underlined.
- Article
- [0]图为在广元市朝天区发现的海南虎斑鳽。[1]广林局供。[2]中新网广元3月15日电。[3]记者15日从四川省广元市野生动物救治中心获悉:近日,该市朝天区东溪河乡的群众发现一只受伤的“怪鸟”引起多方关注,随后,上报到广元市林业部门。[4]后经当地野生动物保护专家鉴定“怪鸟”为世界最濒危鸟类海南虎斑鳽。[5]目前,该鸟在广元市野生动物救治中心喂养。[6]“因为这种鸟极为罕见,也被称为‘世界上最神秘的鸟’。[7]据广元市野生动物救治中心工作人员介绍,经四川省和广元市野生动物保护专家鉴定,该鸟是我国特有的珍稀鸟类、国家二级保护动物海南虎斑鳽,被列为世界最濒危的30种鸟类之一,目前全世界仅存1000余只。[8]海南虎斑鳽未被列入四川省鸟类资源图谱,在四川省发现尚属首次。[9]据百度百科资料显示,海南虎斑鳽是中国特产的鸟类,没有亚种分化。[10]仅见于安徽霍山,广西武鸣、隆安、瑶山、浙江杭州市、临安、天目山,福建西北部邵武、建阳,江西赣州市龙南县九连山自然保护区,广东英德,广东南雄市主田镇瀑布水库和海南白沙、乐东、琼中、五指山等地。其中在海南为留鸟,其他地方为夏候鸟或旅鸟。[11]20世纪二三十年代,在中国浙江、福建、海南等地的山区发现过其踪迹。[12]20世纪60年代初期,华南动物考察队曾在海南作过多次调查,时间长达数年,也仅在中部山区采到了两只标本。[13]此后一直再没有关于该鸟踪迹的报道。
- Summary
- 广元一市民发现受伤“怪鸟”,经鉴定系世界濒危鸟类海南虎斑鳽,全球仅存1000只。
- Label
- 0, 4
- Adequacy
- 1
- Deducibility
- 1
- Article
- 本文总结了十个可穿戴产品的设计原则,而这些原则,同样也是笔者认为是这个行业最吸引人的地方:1.为人们解决重复性问题;2.从人开始,而不是从机器开始;3.要引起注意,但不要刻意;4.提升用户能力,而不是取代人。
- Summary
- 可穿戴技术十大设计原则。
- Human Label
- 5
- Article
- 5月7日,一毕业班55人,仅1名男生。同班女生说他是暖男,只要有力气活的地方他都会出现,一个可顶十个。可惜的是,男生至今未脱单。
- Summary
- 全班55人仅1名男生,被称为暖男却至今单身.
- Comments
- [0]其实主要还是颜值。
- [1]估计太娘了。
- [2]看了他的牙我得到了答案。
- [3]为什么感觉还是和颜值什么的有关。
- [4]哪都有他,这单身理由还不够。
- [5]主要看颜值。
- [6]还是因为现在是看脸的时代,09年学会计的时候班上五十个人,3个男生,一直单身。
- [7]我们全班五十六个人只有一个男生。
- [8]讨厌,大家都是姐妹。
- Article
- 编者按:如果你“不想睡”或者“睡不着”,欢迎继续阅读。这里或许有个文艺片,这里或许有个惊悚片。不知道你会闷到睡着,还是吓得更睡不着。距离1967年《雌雄大盗》(BonnieandClyde)过去已经超过40年,当年饰演邦妮和克劳德的两位主演费伊·达纳韦与沃伦·比蒂在电影里可谓潇洒非凡,尤其是费伊·达纳韦出演的邦妮在电影中穿着长裙在风中端着冲锋枪的造型,早已成为了影史经典的画面之一。两位主演上一次出现在公众的视野里,则造就了奥斯卡历史最大的一场“乌龙”,在颁发最佳影片时,因为会计事务所的失误,导致了二人在台上直接将奖项给错了对象。作为上个世纪美国最为有名的“雌雄大盗”,邦妮和克劳德两人在1930年代美国中南部犯下了多起抢劫以及杀人案件,据记录显示,两人至少杀害过九名警察。一方面二人可谓是声名狼藉,尤其是其残忍杀害公务人员的行径,另一方面身处经济大萧条时期,再经过媒体的大肆渲染,一时之间部分民众甚至将这两位视为“劫富济贫”的罗宾汉人物,甚至当时《雌雄大盗》这部电影也或多或少对这两人的犯罪行为进行了一种美化。而Netflix在3月29日上线的新片《辣手骑警》(TheHighwaymen),则选择了从执法者的角度来讲述这一故事,毫无疑问所有人都知道邦妮与克劳德最终的下场——被德州和路易斯安纳州警方设伏乱枪打死,但其中的细节却并不为人所知晓,包括为何之前警察甚至FBI设下天罗地网都一无所获。显然为了能够对标《雌雄大盗》,《辣手骑警》在演员方面找来了两位好莱坞知名的硬汉凯文·科斯特纳和伍迪·哈里森,正如片名中所提到,二人在电影里饰演的便是已经退休的德州骑警。这一工种原本已经被德州州长所废除,但由于邦妮和克劳德过于猖狂又迟迟无法被抓获,使得州政府有人向州长建议重新找回这些老牌骑警,依靠他们的经验与判断来办案。从类型元素方面来看,《辣手骑警》无疑是标准的侦探片与公路片的杂糅,凯文·科斯特纳和伍迪·哈里森结伴而行,一路寻找着邦妮与克劳德的踪迹,严格来说整部电影相当平淡甚至有些反高潮。尤其是当所有人都知道结局的情况下,显然会更加期待在过程中获得更多的类型化满足,例如双方的斗智斗勇,抑或比较精彩的动作场面,然而这些在这部超过两个小时的电影中几乎都是不存在的,某种程度上两位德州老炮甚至有些狼狈,因为留给他们的线索并不多,同时一路上二人所需要面对的内忧外困却不少。从电影风格的角度来看,讲述同一事件的《雌雄大盗》与《辣手骑警》可谓是一体两面,前者浪漫又疯狂,后者则平淡和苍凉。尤其是当杀人如麻的劫匪被民众视为偶像时,具有老派正义感的退休骑警无论如何也不能理解这个社会为何会变成这样,而他们又执着地想要依靠自己前半生所积累下来的技能与经验来匡扶正义,将恶棍从这荒诞的世界上铲除。导演约翰·李·汉考似乎是一位真人真事改编专业户,之前他执导过两部传记电影《大梦想家》(SavingMr.Banks)与《大创业家》(TheFounder),而《辣手骑警》与他之前执导的作品类似,都有着情节过于平淡的问题,可以说导演每次都能非常工整地完成电影的方方面面,但看完之后却总是缺乏能够让人印象深刻的情节。好在两位好演员在一定程度上为这部平庸之作加分不少,去年在美剧《黄石》中便以牛仔形象视人的凯文·科斯特纳,换上西装便成为了冷酷却坚守正义的德州骑警,而伍迪·哈里森在电影里则更多是一种调节气氛的存在,插科打诨之余,他却与科斯特纳一样都背负着一些不能言说的秘密。令人闻风丧胆的德州骑警,恰恰是因为他们早年违反了执法的程序正义,从一点来讲他们其实与那些罪犯并无区别。相比于从一个新的角度讲述“雌雄大盗”的故事,《辣手骑警》最大的亮点大概在于对当时美国社会现状的展现,一来是正值美国经济大萧条时期,底层民众的生活其实相当困难,这种动荡的经济环境也导致了大量失业人员成为了罪犯;二来则是媒体对于邦妮和克劳德的追捧,两人的事迹屡屡成为了媒体的头版头条,任何举动都被放大,这也使得民众越发崇拜二人甚至愿意替他们掩护从而躲避执法部门的追捕。这种荒诞并非电影故意为之,因为现实恰恰比电影更离奇,在结尾处导演放出了一些历史画面与数据,上万人参加了邦妮和克劳德的葬礼。如果不是《辣手骑警》这部电影,大概鲜有人会知道让“雌雄大盗”丧命的竟然是两位德州退休老头。
- Summary
- 从电影风格的角度来看,讲述同一事件的《雌雄大盗》与《辣手骑警》可谓是一体两面,前者浪漫又疯狂,后者则平淡和苍凉。
We provide some abstractive and extractive baselines for CNewSum.
Since the original summarization metric ROUGE is made only for English,
we follow the method of Hu et al. and map the Chinese words to numbers.
Specifically, the Chinese text is split by characters, and the English words and numbers will be split by space.
For example, “Surface Phone将装载Windows 10” will be transformed to “Surface/phone/将/装/载/windows/10” and then mapped to numeral IDs.
Model | ROUGE-1 | ROUGE-2 | ROUGE-L |
---|---|---|---|
Lead | 30.43 | 17.26 | 25.33 |
Oracle | 46.84 | 30.54 | 40.08 |
TextRank | 24.04 | 13.07 | 20.08 |
NeuSum | 30.61 | 17.36 | 25.66 |
Transformer-ext | 32.87 | 18.85 | 27.59 |
BERT-ext | 34.78 | 20.33 | 29.34 |
Pointer Generator | 25.70 | 11.05 | 19.62 |
Transformer-abs | 37.36 | 18.62 | 30.62 |
BERT-abs | 44.18 | 27.37 | 38.32 |
Analyzing our dataset, we find that the expert editors often perform some reasoning or add external knowledge to make the summary more friendly for the readers.
Thus, we defind two metric to evaluate how much knowledge the model needs to generate the human-like summary.
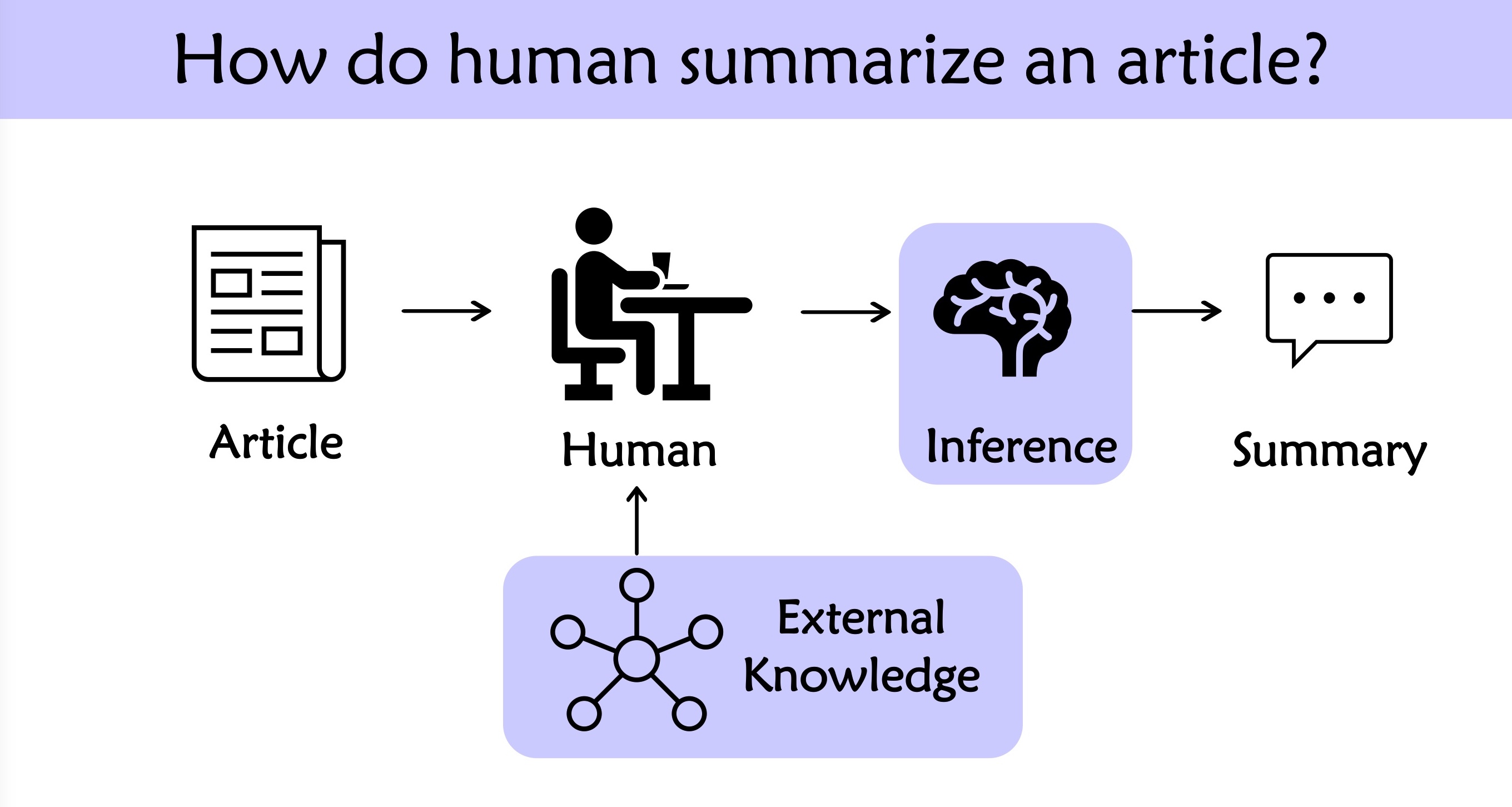
Adequacy
Deducibility
- Unit conversion: 4 kg -> 4000 g
- Number Calculation: 300+1500 -> 1800
- Exchange Rates: 300 dollar -> 1938 RMB
- Name Abbreviation: 武汉大学 -> 武大
We carefully recheck our dataset before the release, and get CNewSum version 2 with updated annotations.
There are about 91.08% examples are adequate and deducible (A = 1 and D = 1), but the rest lack essential information. For 4.11% examples with A = 0 and D = 1, the information can be inferred from the document.
Model | Category | ROUGE-1 | ROUGE-2 | ROUGE-L |
---|---|---|---|---|
Transformer-ext | A=1&D=1 | 33.16 | 19.19 | 27.88 |
A=0&D=1 | 30.89 | 15.60 | 25.38 | |
A=0&D=0 | 28.92 | 14.88 | 23.74 | |
Transformer-abs | A=1&D=1 | 37.54 | 18.85 | 30.83 |
A=0&D=1 | 36.36 | 16.70 | 29.63 | |
A=0&D=0 | 34.73 | 15.95 | 27.52 | |
BERT-ext | A=1&D=1 | 35.05 | 20.67 | 29.62 |
A=0&D=1 | 32.81 | 16.90 | 27.05 | |
A=0&D=0 | 31.07 | 16.57 | 25.72 | |
Bert-abs | A=1&D=1 | 44.51 | 27.76 | 38.70 |
A=0&D=1 | 41.75 | 23.64 | 35.34 | |
A=0&D=0 | 40.18 | 23.34 | 33.60 |